What are the challenges of AI in clinical trials?: AI aka Artificial Intelligence is a term that is well-familiarised in the brain, breath, and body of even the kids out there. AI can be a powerful tool for medical research; when the first time the term Artificial Intelligence was coined back in 1955 during a Dartmouth College conference proposal, the world wasn’t ready for the intervention to be a part of the healthcare sector.
It wasn’t until the early 1970’s when an AI program identified blood infection treatments, that there were slow but sure ripple effects that triggered the change we witness and many experience first-hand today. However, along with the benefits come minor obstacles – which are nothing new for complex systems such as AI.
Of course, it goes without saying that AI has revolutionized not just a single field but numerous fields and one among them is the field of clinical trials. With the advancement of artificial intelligence, there have been several reports of significant challenges popping up with the application of this complex technological advancement into patient care.
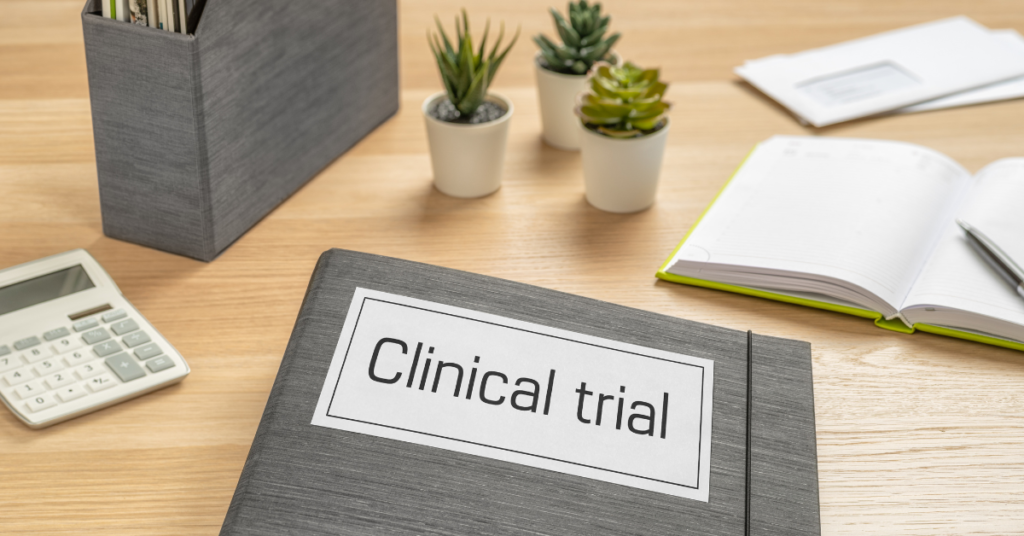
What are the challenges of AI in clinical trials?
The Achilles heel of Artificial Intelligence is simply that it is not human – at the same time, that’s AI’s boon too. While AI algorithms can process large amounts of data quickly, they may struggle to interpret the nuances and context of medical information, leading to potential errors in diagnosis or treatment recommendations. Additionally, AI may lack the ability to consider external factors or make subjective judgments that human clinicians can – makes you think, right?
Here are some of the most commonly reported challenges of AI in clinical trials:
- Transparency: Several reports have highlighted that many AI algorithms, especially deep learning models, operate as “black boxes,” making it difficult to understand how they arrive at specific conclusions.
- Data quality: Inconsistent or incomplete data can lead to unreliable AI predictions – and as mentioned before, AI doesn’t hold total power when it comes to considering the external, minor complexities or making subjective decisions, which in turn can affect the data produced in the end, one of the major challenges of AI in clinical trials.
- Fairness and bias: AI models trained on biased data can perpetuate and amplify these biases, leading to unfair or inaccurate outcomes in clinical trials. In addition to this, making sure that the training data represents diverse populations is crucial to avoid skewed results.
- Cost and implementation: Developing and implementing AI solutions can be expensive, requiring significant investment in technology and expertise. Moreover, another primary challenge of AI in clinical trials is integrating the new AI system with the existing clinical trial infrastructure, which could again be a cost-ineffective solution.
- Ethical considerations: Another important point! Ethical considerations of AI in clinical trials are a subject of discussion, indeed. With the decision-making being transferred from humans to machines (no, this is not Terminator 2….yet), there could be the risk of AI technology interfering with patient autonomy making this one of the worst challenges of AI in clinical trials, if you ask me.
For example, hospitals need to ensure the patients that the confidentiality and security of the information of the patients should be safeguarded to prevent any form of unauthorized access or data breaches.
Now that we’ve gone through the reasons why some are still skeptical of the integration of artificial intelligence in clinical trials, let’s check out the good side.
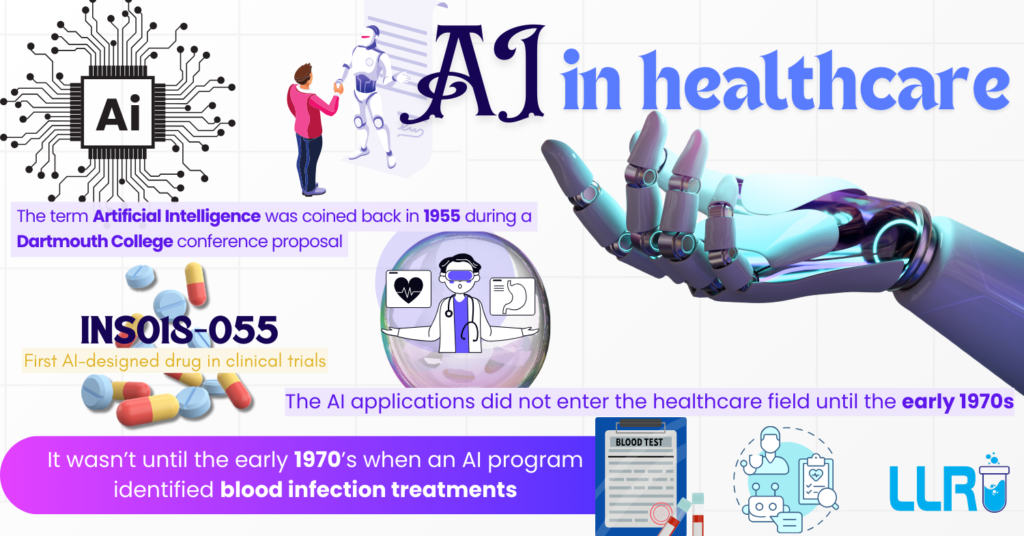
Benefits Of Using AI In Clinical Trials
Yes, there are challenges of AI in clinical trials, we know. But the good must be discussed too, right? Here are benefits of using AI in clinical trials.
Benefit | Description |
Enhanced Patient Recruitment and Retention | AI can analyze vast datasets to identify and recruit eligible participants more efficiently and tailor communication strategies to keep them engaged. |
Optimized Trial Design and Planning | Predictive analytics help design better trials, adaptive trial designs can adjust protocols in real-time, and resources can be allocated more effectively. |
Improved Data Management and Analysis | AI processes and analyzes data in real-time, recognizing patterns and ensuring higher accuracy, which leads to more reliable outcomes. |
Accelerated Drug Discovery and Development | AI speeds up the identification of potential drug candidates and reduces the overall duration of clinical trials, increasing the chances of success. |
Cost Efficiency | AI reduces trial costs by optimizing various processes, minimizing trial failures, and ensuring efficient resource utilization. |
Enhanced Monitoring and Safety | Continuous safety monitoring by AI allows for early detection of adverse events and proactive risk management, ensuring participant safety. |
Regulatory Compliance and Reporting | AI streamlines the preparation of regulatory submissions, improves documentation, and ensures better compliance with regulatory requirements. |
Patient-Centric Approaches | AI enables personalized treatment plans, leading to improved patient outcomes and higher satisfaction by addressing individual needs more effectively. |
So, What Have We Learned Till Now?
While AI holds great promise for revolutionizing clinical trials by improving efficiency, accuracy, and patient outcomes, the challenges of AI in clinical trials need to be addressed. Overcoming issues related to data quality, algorithm transparency, bias, regulatory compliance, and ethical considerations is essential to fully realize the potential of AI in this field.
But – as Geoffrey Hinton, the godfather of AI says, addressing the many challenges, AI can be used to enhance clinical trial processes, leading to more effective and equitable healthcare solutions!
For more information, visit us here!